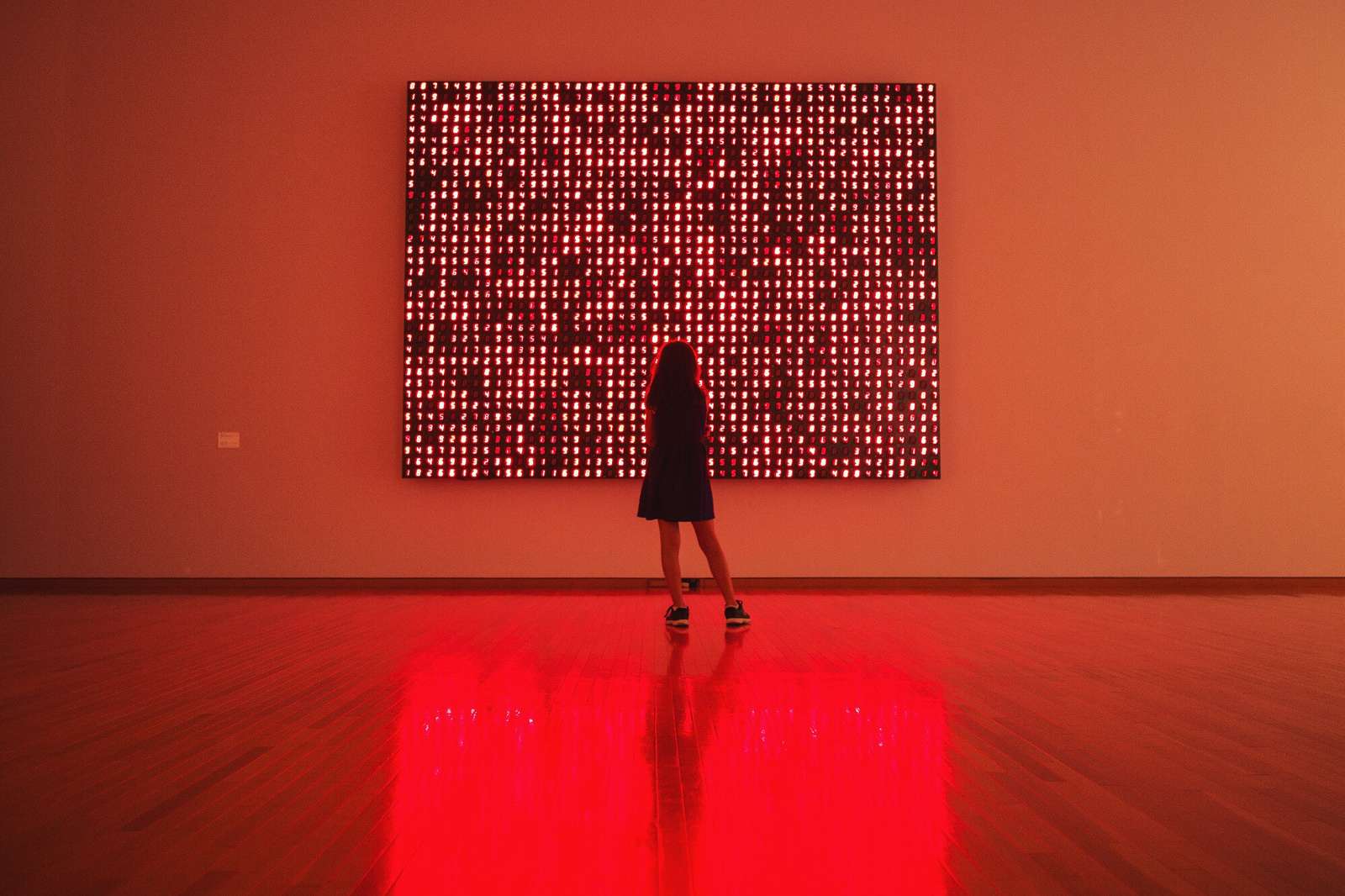
Blog
Data-ness
Some years ago, Stephen Colbert coined the term “truthiness,” something that has the aura of truth because it just feels like the truth.
When it comes to making a point, numbers have a special appeal, no doubt because they appear to offer objective neutrality. But the accumulation of numbers, the use of data, often merely approximates truth, offering “data-ness” as the numerical equivalence of truthiness.
Let me provide a simple illustration. One often sees reports that display the results of a percentage calculation to one or two decimal points. For example, a neighbourhood survey of 128 residents where 72 agreed with a statement could be reported as 56.25% saying “Yes.” While this is mathematically correct, reporting the results to that level of precision suggests a level of accuracy to the overall finding that just isn’t there. How representative are those 128 residents of the target audience? What is the margin of error in this sample? The sheer exactness of the number itself tells us nothing about the rigour of the survey findings. It is the mathematical equivalent of adding attractive graphics to a report that does nothing to advance the argument.
I am often struck by how data frames an issue because as a labour market analyst there is a heavy premium placed on data. Here is a nugget of labour market data: in 2010, a female with a bachelor’s degree working full-time, full-year as a retail salesperson earned less than a male with no educational certificate working as a retail salesperson. The initial shock at this discrepancy is almost eliminated when one explains that males tend make up a higher proportion of workers in such retail sectors as auto sales and electronics stores, which involve higher paying retail positions, while females are more likely to work in clothing and clothing accessory stores, where salespeople are paid less.
This further insight, derived from data, has the tendency to close the discussion: women just happen to work in sectors that are lower-paying. But why is it that occupations or industries that have higher proportions of males tend to be better paying? Data in this instance can help illuminate the question but is of limited help in uncovering the answer. The data feels like it is providing an answer, but it is not providing any understanding of the real, underlying issue.
It is not simply a matter of a quantitative approach drowning out qualitative understanding. The Head Start program in the U.S. provided pre-schooling for low-income children. Initial evaluations showed that Head Start participants scored better on IQ and vocabulary tests after two years of such schooling. But, as time passed, the test score differences shrank, suggesting no extra benefit. The program could not be cancelled, however, because parents were so supportive of it.
As it turned out, evaluations done decades after participation in the pre-schooling showed results that were completely unexpected. Participants in the program were less likely to have been arrested, boys had higher monthly incomes and were less likely to smoke, and girls were less likely to have been put into special education or to have used drugs.
The point for me is, were the parents on to something that was not quite susceptible to measurement at that early stage but which only showed up through intensive, longitudinal tracking?[/alert]
Our reliance on only numbers has gained greater attention in the last few years with the emergence of big data, the ability with massive computing power to gather and analyze huge data sets, for the purpose of identifying patterns and trends. But, in truth, the prominence of data really started rising in the seventies, first in the business sector and migrating subsequently to the public and nonprofit sectors.
What emerged in the seventies was the concept of maximizing shareholder value, the notion that the purpose of a company was to ensure the highest return to its owners, that is, shareholders. This spawned a whole industry of business analysts who engaged in complex calculations to measure and predict rates of return on an investment, creating profit and dividend expectations that publicly traded companies are to meet. These calculations offer the aura of objectivity, because they rest on numbers, in this case typically expressed in terms of dollars or share prices.
But these calculations, seeking the highest return in the shortest period of time, has often led to a discounting of the value of investments that take a longer time to mature, such as research and development or training of workers. A strong argument can be made that this incentive to realize immediate results shortens the time horizons of companies, resulting in just-in-time hiring, use of contract workers, and a disinclination to invest in grooming future talent. Certainly, many a corporate takeover has been paid through the proceeds of “savings” derived from lay-offs — the cost imperative ends up masking what is essentially a balance sheet shift of wage expenditures to dividend increases.
This same mentality of return on investment has infiltrated the nonprofit sector with the drive for demonstrable results. And so we generate data, the more concrete and the more immediate, the better.
Obviously, indicators and outcomes are important — we need to know that the work and money that is put into a project or a program has done some good and that the effort is well-designed and well-targeted. But the premium that is placed on that which can be measured can divert our attention from impacts that have more of a qualitative than quantitative character.
It strikes me that some of the “results” that end up being reported as a consequence of a community sector program or project have that element of “data-ness” – we produce numbers because that is what garners credibility, even if the numbers miss what might be the real benefit of the initiative, like an instance of community building or a set of principles in action, such as seeking social justice or embracing diversity. It would be a shame if in this constant rush to establish the cost of things we were to lose sight of their true value.
At the ONN, we are also seeing the growing importance of data in the work of the nonprofit sector. In fact, we’ve begun looking into what the nonprofit sector needs with our data strategy. As well, the link to data is an important component to our work to develop a Sector Driven Evaluation Strategy, which aims to make evaluation more useful for the nonprofit sector.